No-Reference Quality of Experience Model for Dynamic Point Clouds in Augmented Reality
ACM Mile-High Video 2024
Marriott DTC, Denver, Feb. 11 – 14, 2024
[PDF]
Minh Nguyen (Alpen-Adria-Universität Klagenfurt, Austria), Shivi Vats (Alpen-Adria-Universität Klagenfurt, Austria), Hermann Hellwagner (Alpen-Adria-Universität Klagenfurt, Austria)
Abstract:
In this work, we provide a fine-tuned ITU-T P.1203 model for dynamic point clouds in Augmented Reality (AR) environments. We re-train the P.1203 model with our dataset to get the optimal coefficients in this model that achieves the lowest root mean square error (RMSE). The dataset was collected in a subjective test in which the participants watched dynamic point clouds from the 8i lab database with Microsoft’s HoloLens 2 AR glasses. The dynamic point clouds have static qualities or a quality switch in the middle of the sequence. We split this dataset into a training set and a validation set. We train the coefficients of the P.1203 model with the former set and validate its performance with the latter one.
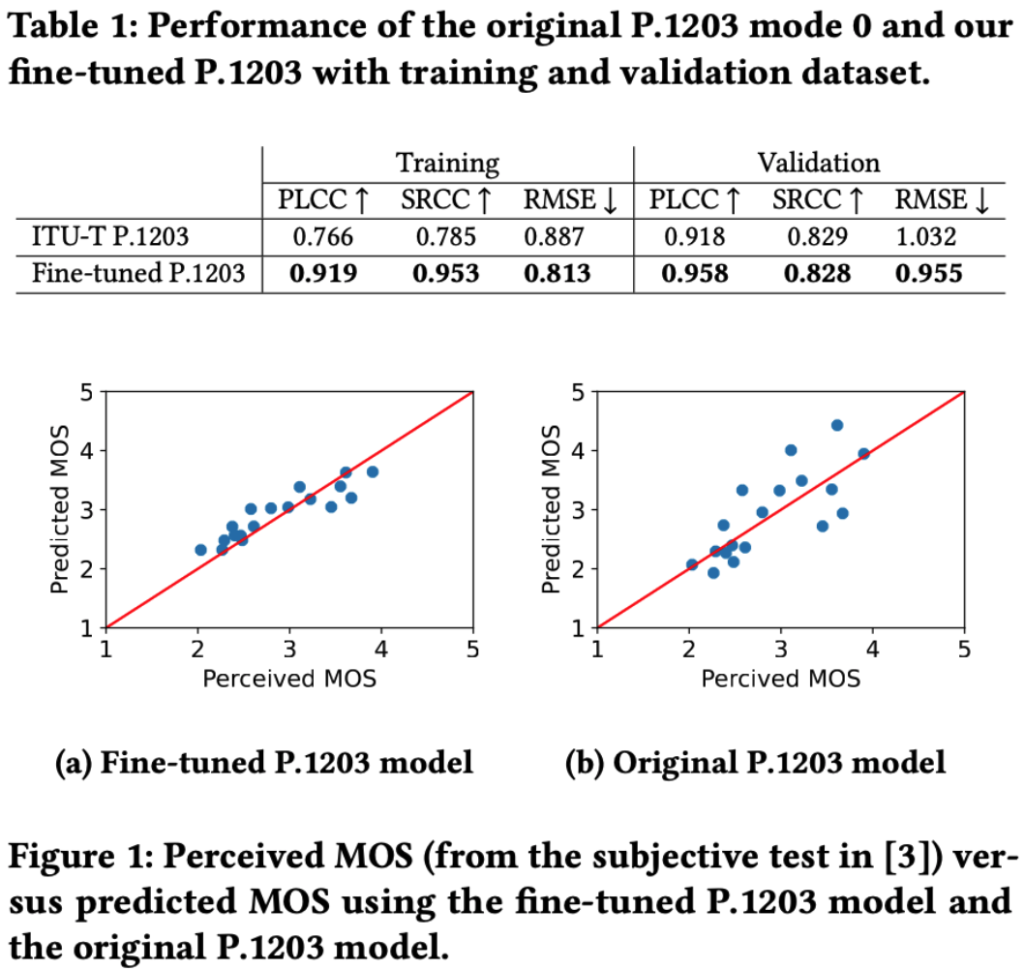
The results show that our fine-tuned P.1203 model outperforms the original model from the ITU. Our model achieves an RMSE of 0.813, compared to 0.887 of the original P.1203 model with the training set. The Pearson Linear Correlation Coefficient (PLCC) and Spearman’s Rank Correlation Coefficient (SRCC) of our fine-tuned model are also significantly higher than that of ITU’s model . These values are more than 0.9 in our model, compared to less than 0.786 in the standard P.1203 model for the training dataset. Taken into account the validation dataset, it can be seen that our fine-tuned model provides a better RMSE = 0.955, compared with 1.032 of the standard P.1203 model. We also achieved a better correlation with the ground truth with PLCC = 0.958 while this metric of the standard P.1203 model is 0.918. The fine-tuned P.1203 model is published in https://github.com/minhkstn/itu-p1203-point-clouds